Analytics
Citizen Data Science: What problems does Einstein solve for our clients?
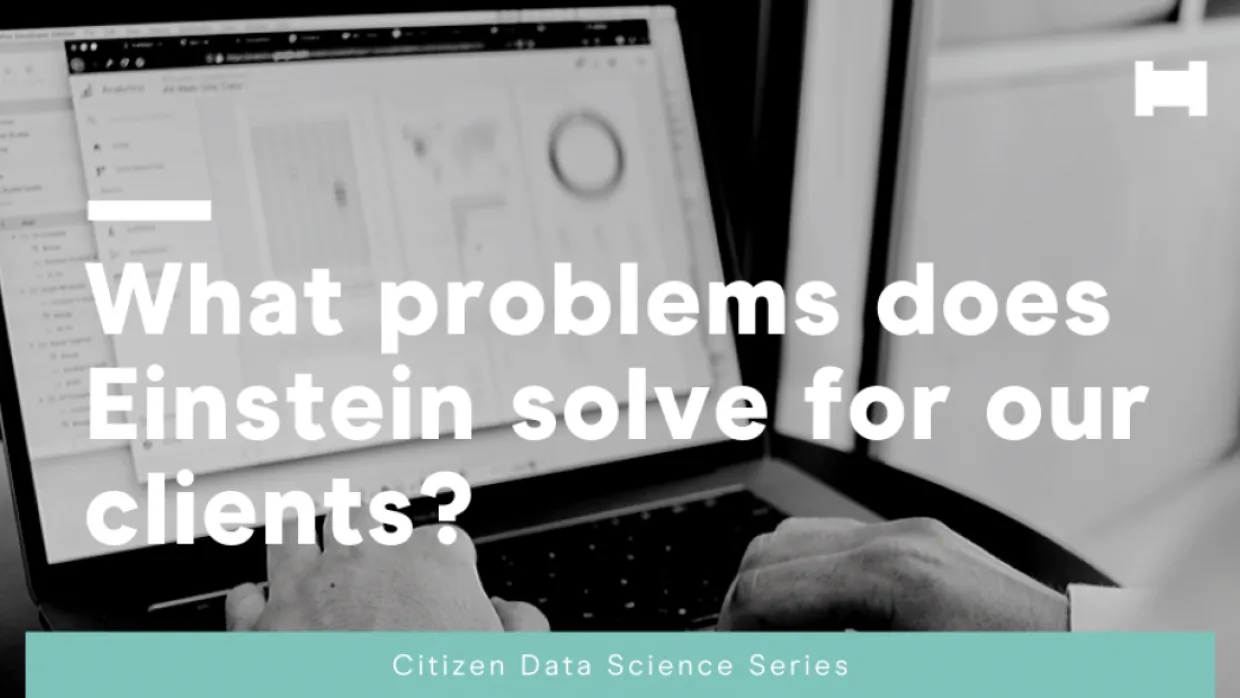
It’s an exciting time to be in the artificial intelligence industry. By 2025, the global AI market size is expected to hit $390 billion—an annual growth rate of 46% from 2019. As auto machine learning continues to develop, more and more companies are turning to AI to solve major business problems.
Thanks to cutting-edge tools like Salesforce Einstein, your computer can blast through oceans of data to unlock greater efficiencies and keep your team firing on all cylinders. But even though auto machine learning can knock out the heavy lifting to free up your team, you still need to figure out the right types of problems to solve. To give you some insight into potential use cases for your business, here’s how Horizontal Digital is implementing Einstein for one of our clients—a global leader in agriculture.
Using predictions to prioritize time and resources
For a business, there’s nothing worse than getting halfway into a project for a customer only to realize you don’t have the technical capability to execute it. If you get to that point, you’ve damaged your reputation and wasted everyone’s time.
But with Einstein AI, you can predict (with around 90% accuracy) whether a project is likely to be technically feasible. The Einstein algorithm looks at thousands of previous projects from two decades worth of data and assigns a winnability score to each new project coming down the pipeline—allowing our client to put their A-Team on the most important projects for their most valuable customers.
This agricultural leader operates on a global scale and works on a wide range of technical projects. Thanks to Einstein AI, they can examine a project and identify why it’s succeeding or failing based on a set of variables. You can also see how much your probability of success increases if you put your best technical scientists on it. Instead of spending time and resources pursuing every possible project with the same intensity, our client can now focus on making strategic decisions and identifying the best opportunities.
Building a bird’s-eye view with operational dashboards
To help this client keep an eye on their accounts more efficiently, we’ve built an operational dashboard that refresh every hour. In a traditional Salesforce environment, you have to regenerate your reports every time your manager requests an update. If you have 15 accounts to keep track of, this can get tedious in a hurry. Plus, there’s no way to view everything at a glance.
But the operational dashboard we built displays charts and percentages that track their goals. It shows them everything—from the very highest level of the company’s performance down to a granular user level. And it just takes a couple clicks to switch from one view to the other.
Understanding how our clients plan to use Einstein
At Horizontal, we endeavor to ask important strategy questions to learn how our clients conduct their business processes. We parse through their answers to better understand how they think about the fields they are inputting and how they're using them on a yearly basis. Einstein is a powerful tool, but every company uses it differently. That's one of the perks—you can optimize it.
Bridging the gap between divisions
For a client of this size and scale, aligning global divisions can be a challenge. For instance, this company’s European division uses Einstein differently than their Asian division. They have different fields. They take a different approach to solving problems and measuring things. To minimize the number of changes and curb confusion, you need to understand the differences between segments and work with them to figure out how things can be more cohesive. Ultimately, this will ensure a smoother implementation.
Exploring future AI opportunities
For this client, the two biggest opportunities for future implementation are natural language processing and image recognition—Einstein Vision and Einstein Language.
For a large scale company like this, Einstein Vision could deliver value in a myriad of ways. For instance, a customer might be buying sugar and think the color is off. Instead of sending a sample to a lab for analysis, Einstein Vision can take thousands of pictures of ways sugar can become tainted or spoiled—as well as thousands of pictures of good quality sugar. Based on these images, the computer builds a learning model. Let’s say the customer’s sugar is contaminated by a certain type of bacteria. Einstein Vision could theoretically pinpoint the issue with 97% accuracy. As the auto machine learning model continues to look at images, it gets more and more accurate.
A cool aspect of Einstein Language is knowledge extraction. For example, let’s say you get an email from a client that asks if you can adjust a quote. In the past, you’d have to manually open the email, switch tabs, click over to that project, scroll down, hit the edit button and type in the new number. With Einstein Language, you just click into Salesforce and it immediately asks if you want to adjust the quote. AI already read your email and predicted your next action.